Dr. Kezunovic and his team presented the latest developments the 2019 Grid of the Future Symposium organized by CIGRE.
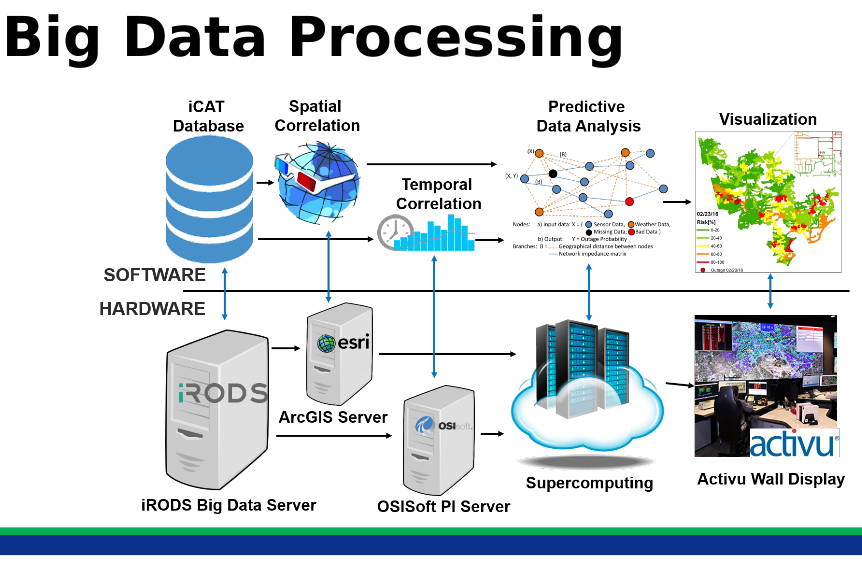
SUMMARY: Loss of electric power leads to major economic, social, and environmental impacts. It is estimated that the Annual economic impacts from weather-related electric grid outages in the U.S. result in as high as $150 billion. Due to the high level of environmental exposure of the electric utility overhead infrastructure, the most dominant cause of electricity outages is weather impact. More than 70% of electric power outages are caused by weather, either directly (e.g., lightning strikes to the equipment, trees coming in contact with lines under high wind speeds), or indirectly due to weather-caused increases in equipment deterioration rates or overloading (e.g. insulation deterioration, line overloading due to high temperature causing high demand). This paper illustrate how the impact of severe weather can be significantly reduced, and in some cases even eliminated, by accurate prediction of where faults may occur and what equipment may be vulnerable. With this predicted assessment of network vulnerabilities and expected exposure, adequate mitigation approaches can be deployed.
To solve the problem, variety of approaches have been deployed but none seem to be addressing the problem comprehensively. We are introducing a predictive approach that uses Big Data analytics based on machine learning using variety of utility measurements and data not coming from utility infrastructure, such as weather, lightning, vegetation, and geographical data, which also comes in great volumes, is necessary. The goal of this paper is to provide a comprehensive description of the use of Big Data to assess weather impacts on utility assets. In the study reported in this paper a unified data framework that enables collection and spatiotemporal correlation of variety of data sets is developed. Different prediction algorithms based on linear and logistic regression are used. The spatial and temporal dependencies between components and events in the smart grid are leveraged for the high accuracy of the prediction algorithms, and its capability to deal with missing and bad data. The study approach is tested on following applications related to weather impacts on electric networks: 1) Outage prediction in Transmission, 2) Transmission Line Insulation Coordination, 3) Distribution Vegetation Management, 4) Distribution Transformer Outage Prediction, and 5) Solar Generation Forecast. The algorithms shows high accuracy of prediction for all applications of interest.